About
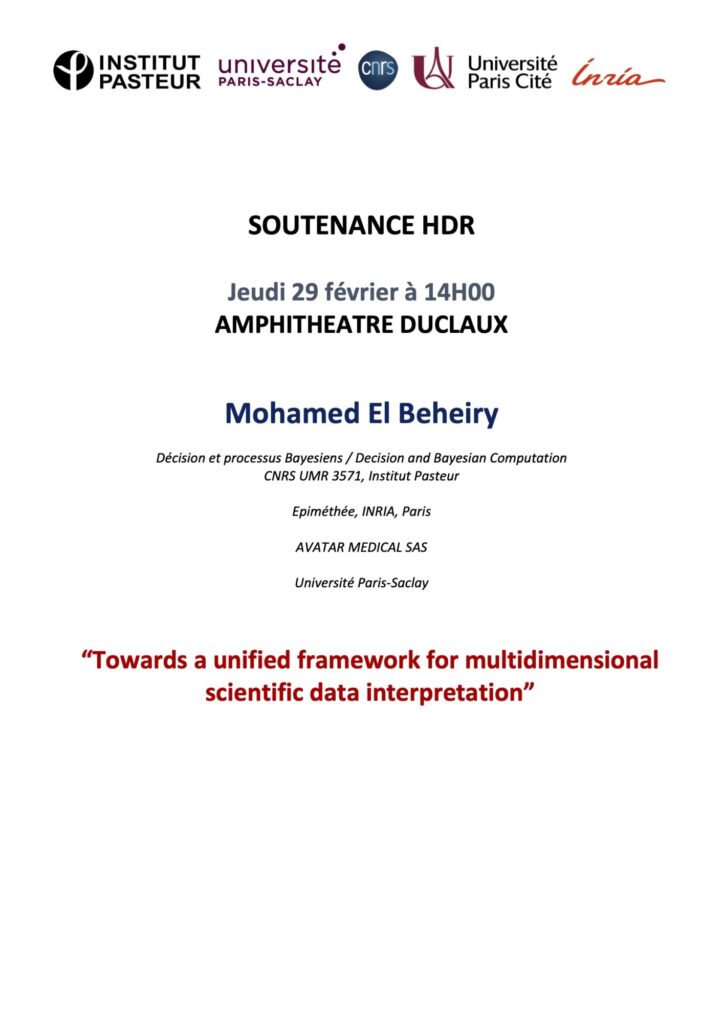
The emergence of new display technologies, analyses and data types encourages the need within scientific and medical communities to streamline the interpretation of multidimensional visual data such as point clouds (\eg localization microscopy, single-particle tracking) and image stacks (\eg confocal microscopy, computed tomography and magnetic resonance imaging). Visual data stakeholders require interactive and adapted means for understanding their data. For this reason, despite the consequential progress of automated algorithms, manual intervention — to different degrees — remains a critical step for data interpretation. Interpretation frameworks meet the needs of users by providing an ensemble of tools, functions and visual readouts to grant insights of the data under study. Ultimately, the end of goal is to validate or invalidate hypotheses and, in so doing, inform decisions being made.
This thesis surveys multiple data interpretation frameworks, with corresponding case studies, where the user intervenes in \textit{human-in-the-loop}-inspired configurations. The discussed tools encompass advanced 3D visualization, virtual reality, Bayesian inference and one-shot learning. Prospective research and development topics to expand the uses, accessibility and performance of these frameworks in scientific and clinical settings are discussed.
Partners
Location
Building: Amphitheatre DUCLAUX
Address: 28 Rue du Docteur Roux, Paris, France