TrackMate (1) is an open-source Fiji plugin initially made for tracking single particles imaged with fluorescence microscopes. It is a user-friendly tool, with a nice interface that lets a user pick among several detection and linking algorithms, and configure them to suit the project needs. In addition to a variety of detectors and tracking algorithms, TrackMate includes advanced visualization and analysis tools, as well as powerful tools for track filtering and curation, giving the user maximal control over the obtained results (Figure 1). Since its publication in 2017, it enjoyed some popularity and a decent user base, with an estimated 35k users and 1,800 citations of the first paper.
We started its development years ago in the optical microscopy core facility of Pasteur (PBI, then PFID and Imagopole) as a tool for a quantitative study on the phototoxic impact of different imaging modalities (2). Since then, it has been used, augmented and refined through countless user projects in the PBI or IAH core facilities (3–8). It has interoperability tools with MATLAB, Python and Icy. It is made to be easily and quickly extended so that it can be adapted to various samples and studies. For us in the facility, it is mainly a software platform that allows us to deliver customized tools for user projects in a timely manner.
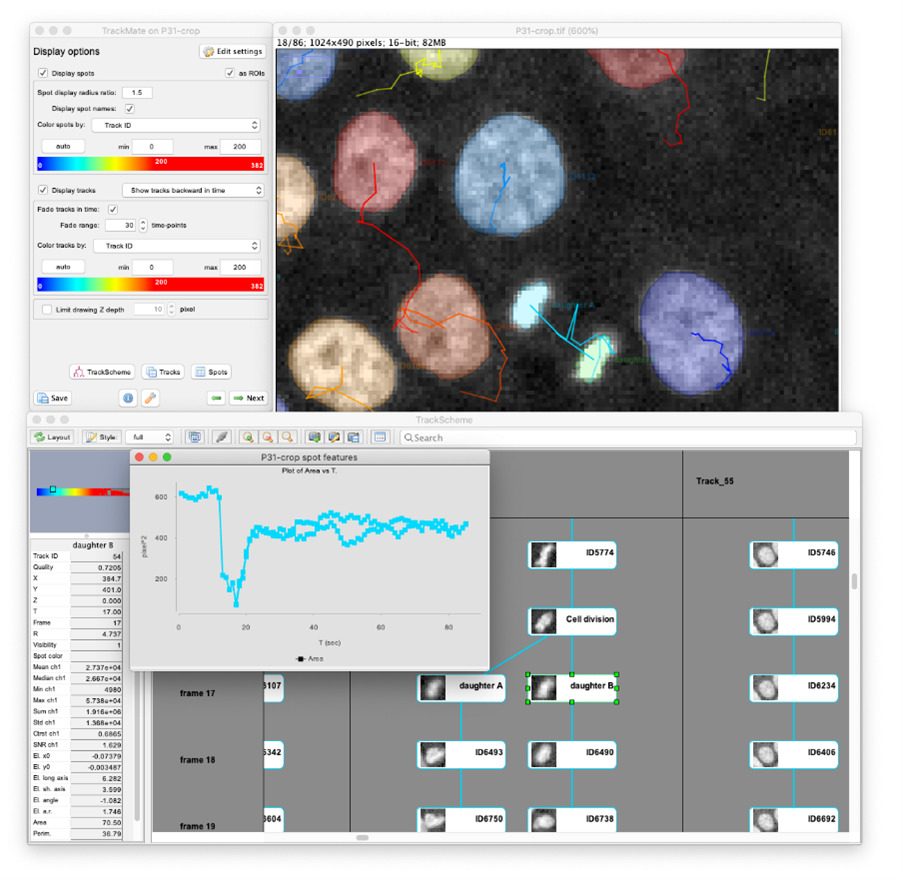
Very recently, a user-project with the unit of Guillaume Duménil fostered its development again. The previous version of the TrackMate had excellent detectors for the detection of light blob-like objects on dark background using the Laplacian of Gaussian (LoG) or Difference of Gaussians (DoG) detectors. While these detectors perform well for fluorescent images, they still struggle when trying to detect textured, irregular objects, or objects imaged in transmitted-light microscopy (bright-field, phase contrast, DIC). As image segmentation, machine-learning (ML) and deep learning (DL) methods have taken a massive leap forward in the last few years, it was time to incorporate some of these features into TrackMate. The Covid-induced lockdowns gave us a great opportunity to rewrite TrackMate almost from scratch, to make it possible to integrate these algorithms. We collaborated with the team of Guillaume Jacquemet (University of Turku, Finland) for the code and incorporated DL and ML segmentation algorithms, such as StarDist, cellpose, ilastik and Weka to TrackMate, making it possible to apply custom-made segmentation algorithms to a specific dataset directly.
This integration proved to be successful and to considerably widen the range of problems that TrackMate can tackle (2). For instance, DL algorithms offer a very robust segmentation of cells imaged in transmitted light, which is very difficult and error prone with classical methods. Thanks to their integration in TrackMate, we can now perform cell tracking and lineaging on label-free stem cells, using transmitted light imaging. This considerably lowers the difficulty and failure-rate of these experiments, while permitting long-term time-lapse imaging as transmitted light displays a low phototoxicity and does not require the transformation of cells for imaging. We can also segment and track cells in dense and compact arrangement, following single cells migration during tissue movement or in wound healing experiments. And TrackMate gives us all the quantitative morphology information on the cell shape, that can be used in tracking for instance, when building bacterial lineages. These new capabilities as well as several others were the subject of our latest article published this month in Nature Methods (9).
For the Pasteur campus, TrackMate will now enjoy some time being a tool that support your projects, instead of being the focus of the team development. We hope you will find it useful for your Research and that its capabilities will inspire and facilitate new quantitative approaches to the biology you study!
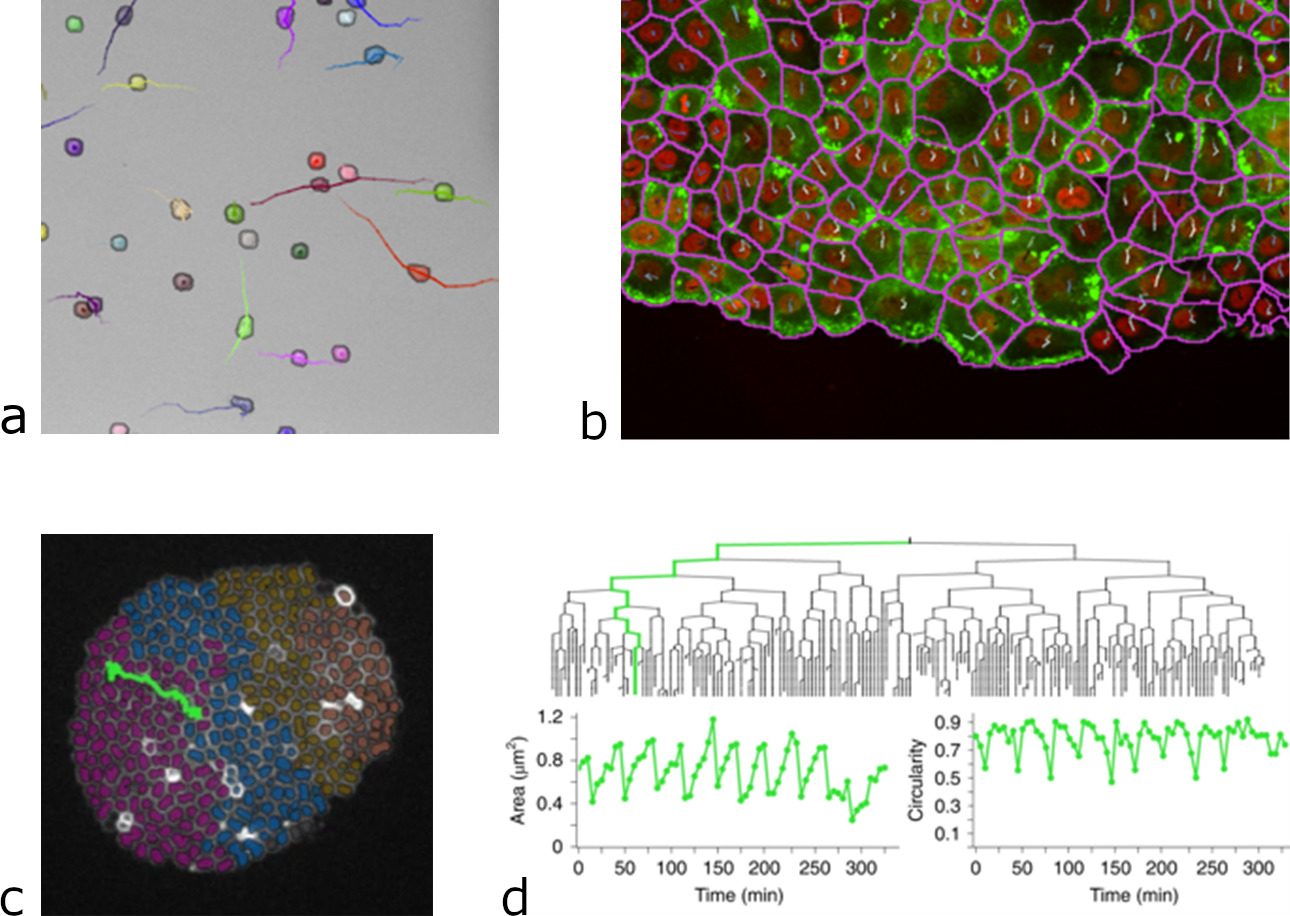
- Tinevez JY, Perry N, Schindelin J, Hoopes GM, Reynolds GD, Laplantine E, et al. TrackMate: An open and extensible platform for single-particle tracking. Methods. 2017 Feb 15;115:80–90.
- Tinevez JY, Dragavon J, Baba-Aissa L, Roux P, Perret E, Canivet A, et al. A quantitative method for measuring phototoxicity of a live cell imaging microscope. Methods Enzymol. 2012 Jan 1;506:291–309.
- Tarantino N, Tinevez JY, Crowell EFEF, Boisson B, Henriques R, Mhlanga M, et al. TNF and IL-1 exhibit distinct ubiquitin requirements for inducing NEMO–IKK supramolecular structures. J Cell Biol. 2014;204(2):231–45.
- Arena ET, Campbell-Valois FX, Tinevez JY, Nigro G, Sachse M, Moya-Nilges M, et al. Bioimage analysis of Shigella infection reveals targeting of colonic crypts. Proc Natl Acad Sci. 2015 Jun 23;112(25):E3282–90.
- Dejarnac O, Hafirassou ML, Chazal M, Versapuech M, Gaillard J, Perera-Lecoin M, et al. TIM-1 Ubiquitination Mediates Dengue Virus Entry. Cell Rep. 2018;23(6):1779–93.
- Wolff C, Tinevez JY, Pietzsch T, Stamataki E, Harich B, Guignard L, et al. Multi-view light-sheet imaging and tracking with the MaMuT software reveals the cell lineage of a direct developing arthropod limb. eLife [Internet]. 2018 Mar 29;7. Available from: https://elifesciences.org/articles/34410
- Samassa F, Ferrari ML, Husson J, Mikhailova A, Porat Z, Sidaner F, et al. Shigella impairs human T lymphocyte responsiveness by hijacking actin cytoskeleton dynamics and T cell receptor vesicular trafficking. Cell Microbiol. 2020;22(5):e13166.
- Tinevez JY, Herbert S. The NEMO Dots Assembly: Single-Particle Tracking and Analysis. In: Miura K, Sladoje N, editors. Cham: Springer International Publishing; 2020. p. 67–96. Available from: https://doi.org/10.1007/978-3-030-22386-1_4
- Ershov D, Phan MS, Pylvänäinen JW, Rigaud SU, Le Blanc L, Charles-Orszag A, et al. TrackMate 7: integrating state-of-the-art segmentation algorithms into tracking pipelines. Nat Methods. 2022 Jun 2;1–4.