Context & Objectives
Genome-wide association studies (GWAS) – i.e. systematic study of the link between millions of genetic variants and a trait in a sample of individuals – have been central to decipher the genetic factors of human traits. As of today, GWAS have reported over 150K associations for thousands of heritable traits (e.g. height, type 2 diabetes or cardiovascular diseases)[1]. This incredible amount of data has notably been used to assess the genetic risk – i.e. to compute a Polygenic Risk Scores (PRS) – of developing human chronic diseases[2]. Yet, the predictive performances reached by PRS have been hampered by the convoluted genetic architecture of these complex traits. State of the art PRS method do not include functional annotation as part of their calculation. Our group has developed an internationally recognized expertise in multi-traits analysis methods –i.e. joint statistical tests of genetic variants on several complex traits- and their associated computational tools[3]. In particular, our recent work focused on how multi-traits analysis can be used to uncover functional pathways. In this context, we propose to leverage our expertise in functional annotation to: 1) improve the predictive power of PRS, 2) provide the repartition of the genetic risk in functional pathways for each individual.
A primary target for our ongoing multi-trait genetic analyses is the genetic of metabolism and its link to diseases. Indeed, cholesterol and glucose plasma concentration are causally implicated in three of the deadliest chronic diseases globally[4] (coronary heart disease, Strokes and diabetes). Hence, the utmost importance of better understanding the genetic of metabolism to improve and personalized treatment.
The trainee will use recent GWAS on metabolic traits and the UKBIOBANK cohort[5] to improve polygenic risk scores. The trainee will be asked 1) to build upon a decomposition of the genetic effect in functional pathways to construct a PRS, 2) to apply and assess the PRS accuracy on the UKBIOBANK cohort 3) to observe if different types of genetic risk profile can be distinguished in UKBIOBANK individuals. The trainee will review literature to apply state of the art methods.
During his/her internship, the trainee will benefit from the stimulating scientific environment of the Pasteur Institute including regular computational biology seminar and large group of bioinformatician and biostatistician to exchange with.
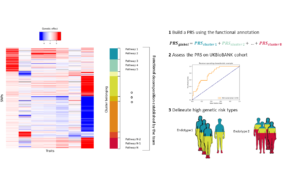
QUALIFICATIONS
The position requires advance knowledge in statistics and computer sciences. The applicants should therefore have substantial educational background in statistics/biostatistics, computer Science or other relevant disciplines. Prior knowledge in biology would be an asset. The call specifically addresses master 2 students and 3rd year engineer school students.
ADDITIONAL INFORMATION
Interested applicants should send their curriculum vitae, a grade transcript, a brief cover letter, and contact information from at least one referee (e.g. teacher or previous internship mentor) to Dr. Hanna Julienne (hanna.julienne@pasteur.fr). More information about our group and the computational biology department can be found here https://research.pasteur.fr/en/team/statistical-genetics/ and here https://research.pasteur.fr/en/department/computational-biology/ .
[1] https://www.ebi.ac.uk/gwas/diagram
[2] https://www.nature.com/articles/s41588-018-0183-z
[3] http://jass.pasteur.fr/index.html
[4] https://www.who.int/news-room/fact-sheets/detail/the-top-10-causes-of-death
[5] https://www.ukbiobank.ac.uk/about-biobank-uk/
[gview file=”https://research.pasteur.fr/wp-content/uploads/2020/09/research_pasteur-m2-internship-leveraging-functional-annotation-to-improve-genetic-risk-assessment-context-amp-objectives-sujet-stages-clustering-prs-2020.pdf”]